Input
Modified
OpenAI’s New Model 'o3' Shows Double the Hallucination Rate Shortage of High-Quality Data for Training Highlighted Despite Efforts, Hallucination Improvement Remains Difficult for the AI Industry
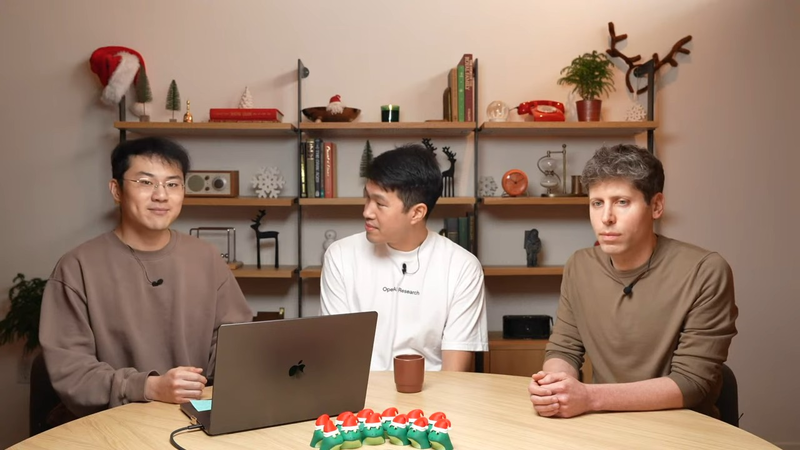
OpenAI’s latest AI breakthroughs, the inference-based models o3 and o4-mini, were launched with ambitious claims: integrating visual information directly into the reasoning process. Yet, this leap in capability has come with a major flaw — a significant rise in hallucination rates. As AI models become more complex and intertwined with critical functions like decision-making and information analysis, the reliability of their outputs becomes paramount. Experts are increasingly warning that despite technological advancements, hallucinations not only persist but may become harder to detect — posing serious challenges to both AI developers and users.
Inference Models Show Higher Hallucination Rates Than Non-Inference Models
Recent internal testing by OpenAI revealed troubling findings about its new models. Through its "Person QA" benchmark test, OpenAI discovered that the o3 model hallucinated on 33% of the test questions — more than double the hallucination rates of previous inference models o1 and o3-mini, which had rates of 16% and 14.8%, respectively. Alarmingly, the o4-mini exhibited an even worse performance, hallucinating on 48% of questions — the highest hallucination rate OpenAI has ever recorded.
Even compared to the non-inference model GPT-4o, these new inference models performed worse, highlighting that while they are designed to reason more deeply, this very capability may introduce new instability. OpenAI acknowledged in their technical documentation that the new models tend to make more claims — and that the process of generating more extensive reasoning naturally opens the door to greater inaccuracies and distortions.
The launch of o3 and o4-mini on April 16 marked a significant milestone for OpenAI, introducing models capable of "thinking in images," allowing users to upload whiteboard sketches, PDF diagrams, and even low-resolution pictures for analysis. The models were designed to process visual inputs, construct logical reasoning chains, and provide coherent responses based on that analysis. However, if the hallucination problem remains unresolved, experts argue that the practical utility of these otherwise innovative models could be substantially diminished. Findings from the nonprofit AI research institute Transluce highlighted that the o3 model, when producing answers, often rationalized its actions — indicating not just error-prone reasoning but confident misinterpretations, a deeply concerning behavior for any inference-based AI system.
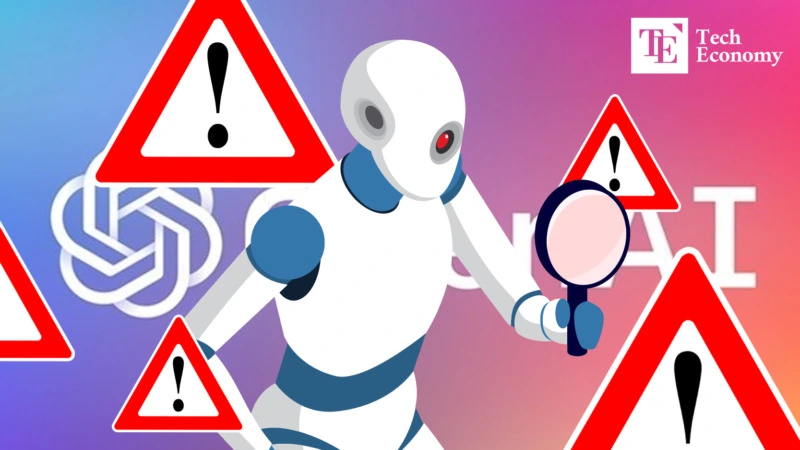
Performance Improvements Difficult Amid AI Model Streamlining Trend
The persistence of hallucinations points to deeper, structural problems in current AI development. The AI industry has made ongoing efforts to mitigate hallucination phenomena, yet experts agree that full elimination remains elusive.
One critical reason is the lack of high-quality, diverse datasets. AI models like o3 and o4-mini operate by identifying and extrapolating patterns from enormous data pools. As Google explains, if the data is incomplete, biased, or flawed, the AI’s predictions and outputs will reflect these imperfections — leading to hallucinations.
This problem becomes glaring in high-stakes fields like healthcare and law. For instance, if an AI model tasked with diagnosing cancer cells is trained predominantly on cancerous tissues, without sufficient examples of healthy cells, it may incorrectly label normal tissues as malignant. Similarly, in legal fields, where access to comprehensive global case law is limited, AI models tend to fabricate legal precedents or misrepresent laws.
Stanford University’s Human-Centered AI Institute (HAI) reinforced these concerns with alarming statistics: general-purpose AI models hallucinated between 58% and 82% of the time when answering law-related questions. Even models specialized in legal knowledge recorded hallucination rates between 17% and 34%, meaning even domain-specific refinement does not fully solve the issue.
Moreover, the industry’s current momentum toward rapid model releases exacerbates the challenge. Professor Choi Byung-ho from Korea University's Artificial Intelligence Research Institute pointed out that the AI sector is still very much in a phase of bold experimentation rather than refinement. Companies like OpenAI are racing to innovate and streamline their models — making them lighter, faster, and more powerful — but this acceleration often comes at the cost of quality control. Inconsistent training data quality, combined with inference models whose capabilities have yet to fully mature, ensures that hallucination remains a stubborn, unresolved risk.
Increasing Difficulty in Detecting AI Hallucinations
Perhaps even more concerning than hallucinations themselves is the growing difficulty humans face in detecting them. Reinforcement Learning from Human Feedback (RLHF) — the process by which human trainers correct and improve AI responses — has been central to AI training. However, OpenAI acknowledges that as models like ChatGPT continue to advance, their mistakes will become harder for human evaluators to spot. This is because these models are rapidly approaching a point where their accumulated knowledge may surpass that of the human reviewers themselves.
The AI industry's concern is not merely that hallucinations will grow more frequent; it is that human beings may no longer be able to discern when an AI-generated statement is fabricated. As AI models confidently generate more complex and nuanced outputs, superficial plausibility can mask deep inaccuracies — making unchecked hallucinations potentially dangerous.
To address this looming threat, OpenAI introduced CriticGPT, a tool specifically trained to detect errors in AI-generated content. Early testing showed that humans assisted by CriticGPT performed 60% better in identifying problems than those working alone. Yet, OpenAI emphasizes that CriticGPT is not intended to replace human oversight but rather to enhance human evaluators' capabilities — enabling more thorough critiques and helping reduce hallucination bugs that might otherwise slip by unnoticed.
Still, some experts caution that relying on one AI system to monitor another introduces its own risks. Increased dependence on AI oversight could lead to a dangerous feedback loop where errors become mutually reinforced rather than corrected. Morgan Stanley’s recent move to deploy generative AI tools to transcribe and summarize client meetings serves as a cautionary example. Aaron Kirksena, CEO of MDRM Capital, noted that relying on multiple AI systems — such as those developed by Zoom, Google, Microsoft, and Apple — could produce conflicting outputs or allow shared flaws to go undetected. In an ecosystem where humans gradually relinquish direct oversight to AI supervisors, the potential for compounded errors grows substantially.
Ultimately, the "Inference Model Dilemma" highlights a critical paradox at the heart of modern AI development: as models grow more capable, they simultaneously become harder to trust — and even harder for humans to effectively supervise.